Decarbonizing the industrial sector is a growing priority. To help produce models with high-quality data that inform policy, transparency and dialogue should be fostered among modeling colleagues, policymakers, and the modeling community.
Greenhouse gas emissions from the heavy-industry sector of the economy commonly are referred to as “hard to abate,” especially in discussions about achieving deep decarbonization (i.e., completely, or nearly completely, eliminating emissions) across various industrial subsectors such as iron and steel or cement. These industrial emissions are hard to abate for a few reasons. First, industry subsectors tend to involve high-temperature, energy-intensive processes that are difficult to replicate with electrification or less energy-intensive processes, given the limitations of existing technologies. These subsectors also often produce carbon dioxide emissions as a byproduct of manufacturing (e.g., cement). Second, the technologies that are necessary to achieve deep decarbonization of these industries often are theoretical, unproven, or cost prohibitive. In the power and transportation sectors, on the other hand, low-carbon options have seen significant innovation over the past few decades and are relatively straightforward.
The complexities in decarbonizing these hard-to-abate industrial sectors also make it hard to predict how effective various policies can be in encouraging decarbonization and the implementation of advanced technologies. The current set of models that can predict changes in industrial energy demand and associated emissions over time are useful, but these models have limitations. Take an example of a model which suggests that a given policy would have little effect on future emissions. Does this result really mean that the policy is ineffective? Or does the result suggest that the model does not accurately reflect the mechanisms through which the policy could achieve emissions reductions?
Challenges in Modeling Industrial Decarbonization
Researchers at Resources for the Future (RFF) have done a deep dive into various approaches for industrial-sector modeling over the past year and a half. We published a report (with colleagues from our partner institution, the RFF-CMCC European Institute on Economics and the Environment) that reviews the strengths and weaknesses of existing approaches to industrial-sector modeling. We also hosted a private workshop that focused on identifying the challenges involved in modeling policies and pathways for deep decarbonization across energy-intensive industrial sectors.
Each energy-intensive subsector of industry—such as steel, cement, aluminum, chemicals, and pulp and paper—has its own unique approaches to decarbonization and faces its own unique challenges. Approaches to decarbonizing these sectors include switching sources of energy (i.e., replacing technologies and processes that are powered by fossil fuels with alternatives powered by electricity, green hydrogen, or biofuels), improving energy efficiency in production processes, developing new processes that emit less greenhouse gases, using materials more efficiently, implementing carbon capture and storage, reducing waste, and recycling products. Any of these methods alone in any given sector likely would not be enough for deep decarbonization, and each specific sector likely would need to use a combination of these approaches and use them to varying degrees. Any modeling framework to address deep decarbonization in industrial sectors therefore must be able to include a variety of options and approaches to decarbonization.
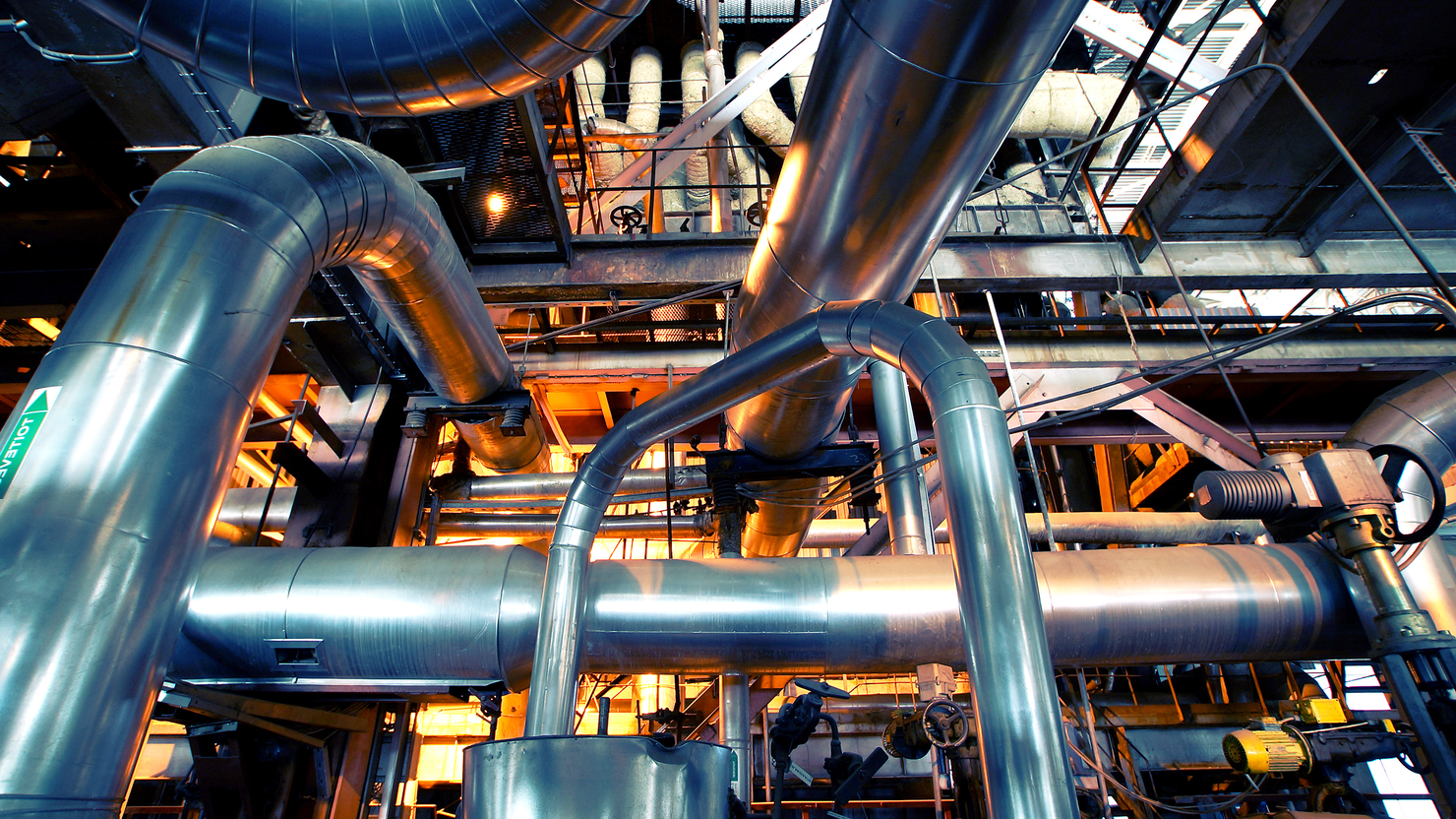
Further complicating industrial-sector modeling are data challenges. Attaining the appropriate data for modeling these sectors can be difficult. The availability and detail of data vary across countries, and how easily that data can be compared to the data from other countries, also can vary wildly. Even within the United States, it can be difficult to determine the precise production processes used (and related energy intensities) across different facilities, let alone make reasonable predictions of the uptake of decarbonizing technologies. Modeling the adoption of advanced technologies requires predicting the costs and performance of unproven technologies that don’t currently exist at scale, which can force modelers to rely on probabilistic forecasts of energy-technology costs that often tend to be pessimistic.
As we worked on our deep dive into industrial-sector decarbonization, we realized that perhaps the biggest challenge for industrial-sector modelers is that, despite the numerous teams working on these kinds of modeling frameworks, modelers often work in silos. Little to no collaboration or data-sharing occurs between different modeling teams. Further, modelers often are detached from policymakers who are looking to develop new policy solutions for decarbonizing industrial sectors; in other words, modelers may be answering questions that are not as relevant to current policy discussions as they should be.
Fostering Collaboration
To foster collaboration among modeling teams, and between these groups and policymakers, RFF invited key modelers, policymakers, and other interested stakeholders to a private workshop in May 2023. The workshop followed the Chatham House Rule: participants were free to use information shared at the workshop as long as the source of the information remained anonymous. At the workshop, the group of participants agreed that sharing ideas more freely could help with some of the more difficult aspects of industrial-sector modeling; for example, how to approach international trade, integrate clean energy technologies into models, and solve other methodological or analytical problems.
Another consensus was that sharing data between teams also could help address data availability issues. Because different teams have access to different databases and data sets, a process and a location for sharing that data between teams would be incredibly beneficial to all teams that model the industrial sector. Of course, this idea raises the question of how modelers can collaborate on data, and who would fund and maintain an industrial-sector database that could be used across different modeling platforms.
Further complicating industrial-sector modeling are data challenges. Attaining the appropriate data for modeling these sectors can be difficult. The availability and detail of data vary across countries, and how easily that data can be compared to the data from other countries, also can vary wildly.
At the workshop, participants also identified modeling international trade as a tricky topic, especially as it relates to modeling unilateral climate policy or trade restrictions such as border adjustment mechanisms, which impose fees on imported products based on the greenhouse gas emissions associated with a given product. Participants posed some relevant questions. What’s the better approach to model international trade: linking industrial-sector models with trade models, or accounting for trade within the industrial-sector model itself? Would we get the most accurate projections by using models for international trade that already exist—or should we develop new models for international trade that are specific to industrial subsectors, and then link these new models to industrial-sector models that are tailored to specific countries?
Finally, we identified a need for continued dialogue between policymakers and modeling teams. Policymakers need to know which models are or are not well suited to answer specific questions, and modelers need to know the types of policies that are under consideration and related questions that are being asked. Through continuing conversations, modeling teams can help policymakers and stakeholders understand the outcomes of different policy choices in terms of emissions, costs, impacts on various demographic groups, and equity among communities. This information can both improve decisions and facilitate consensus on the most effective policies across stakeholders and the general public.
In the coming year, RFF will host similar convenings with familiar and new participants to build a community of industrial-sector modelers that jointly share data, research agendas, and best practices; help each other address common challenges; and align their research to answer the most policy-relevant questions. This growing community can help policymakers develop policies for industrial decarbonization that are effective, efficient, and fair.