The current land-based pollution monitoring network in the United States fails to account for fine particulate matter pollution across much of the country. Satellite data can help fill in the gaps.
Clean air is invisible. But sometimes polluted air is, too—even according to the network of monitors in the United States that’s specifically designed to tell us what we can’t see.
The air pollution monitoring network run by the US Environmental Protection Agency (EPA) has shown that air pollution in the form of fine particulates (termed PM2.5, which is particulate matter that has a diameter of 2.5 microns or less) violates this pollutant’s air quality standard for 23.3 million people. But former RFF Fellow Daniel Sullivan and I have found that EPA’s national network of land-based air quality monitors is so thin that it misses many other areas of the country that also violate the standard. Our work shows that EPA and its land-based monitoring network have failed to identify 54 counties and another 25 million people in the United States who live in areas that violate these air quality standards.
Satellite data can help with the shortcomings of our land-based monitoring network. Satellites now are pervasive and accessible; they can provide comprehensive, up-to-date, high-resolution data to estimate PM2.5 concentrations across the country. With all these data coming from satellites, it is time for some serious re-thinking of Title I of the Clean Air Act (CAA) and the regulation concerning how areas are designated as meeting or violating the air quality standard.
How Has the Current Monitoring System Gotten Things so Wrong?
One big downside to this system is that the land-based monitoring network is sparse. As Daniel Sullivan and I have shown, the majority of US counties lack monitors altogether. Of 3,100 counties in the United States, only 651 (21 percent) have any PM2.5 monitors at all. Among those 651 counties as of 2015, about 48 percent had a single monitor, 24 percent had two monitors, and only 29 percent had three or more. Furthermore, readings at an air pollution monitor do not necessarily represent the full range of concentrations across areas as large as a county.
Why are monitors so sparse? One reason is that they are expensive. EPA identifies specific “federal reference monitors” as appropriate for monitoring air pollution consistent with the CAA (as documented in 40 CFR part 53). EPA says that the cost of buying the approved monitors varies between $15,000 to $50,000, with additional operating costs labeled as “expensive.” However, “expensive” is relative, and other options exist. States have ready access to a suite of air quality sensors that can be purchased for under $2,500. These sensors are meant to be relatively cheap and to supplement, rather than replace, devices that follow federal reference methods for sampling and analyzing ambient air. As we recommend below, satellite data could be used similarly, but with better results.
With a limited number of monitors in an area, their placement is critical. Even more so if pollution concentrations have steep spatial gradients and vary a lot with weather conditions, economic activity changes from day to day, and economic growth continues over the longer term. All these conditions generally hold.
Conventional wisdom is to assume that the concentrations registered by the required monitoring network are representative of concentrations throughout the area in question, and good monitor placement is critical to this assumption. EPA has rules for where monitors must be placed (per 40 CFR part 58 of the CAA), and the EPA administrator has ultimate authority to approve a monitor network. But the rules explicitly (and understandably) balance data needs with government resources.
While more technical documents may help govern monitor placement, the main document noted in the “Network Design Criteria for Ambient Air Quality Monitoring” appendix of the related federal regulations code outlines minimum monitoring requirements only. For example, only three monitors are required for PM2.5 in designated metropolitan areas over 1,000,000 people and with design values near or exceeding the CAA’s established National Ambient Air Quality Standards. Minimum requirements mandate one near a roadway and one in an area of expected maximum concentration at the neighborhood or urban scale. The rules also designate that monitors should not be located “in the immediate vicinity of any single dominant source [of emissions].”
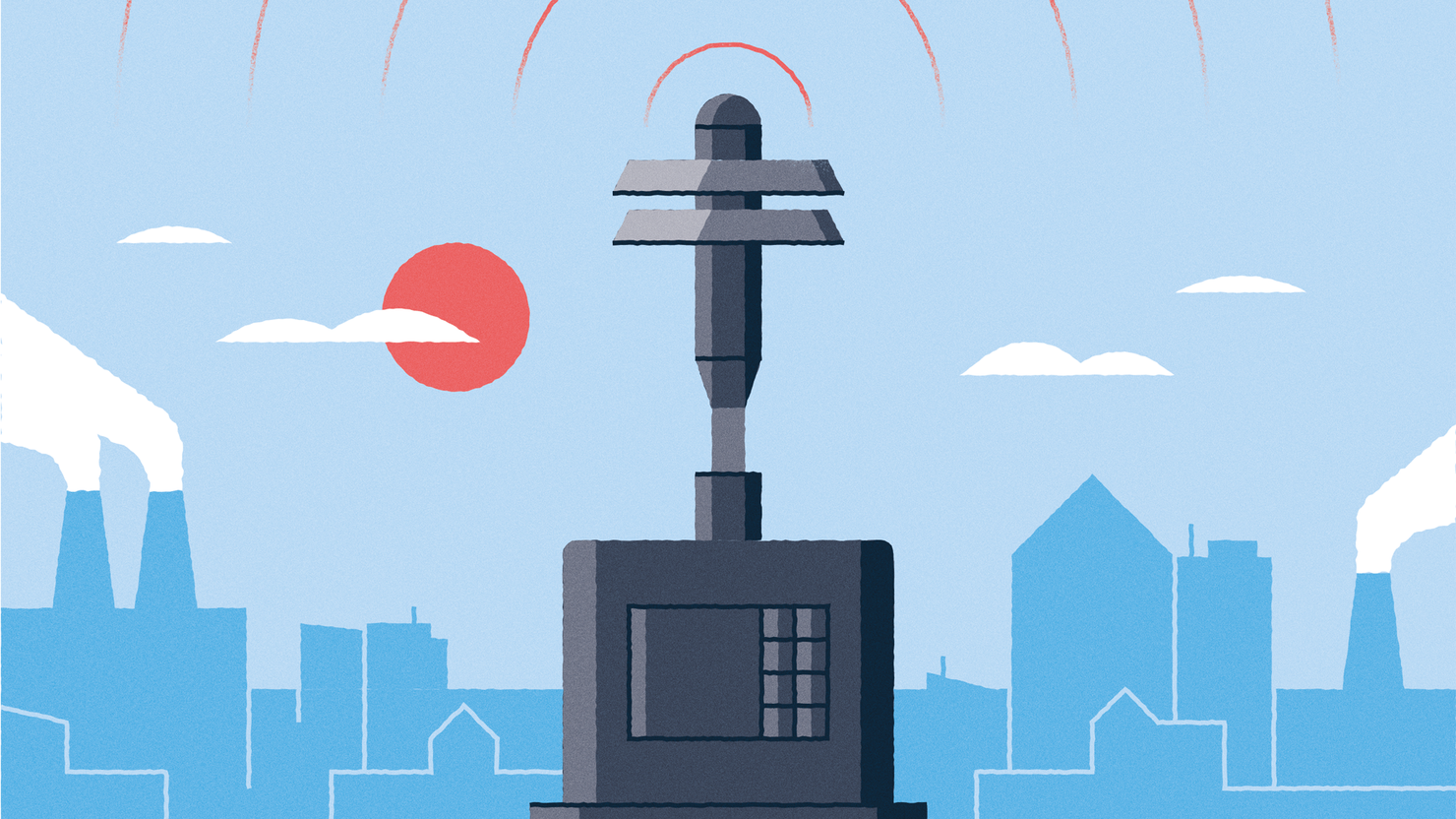
Thus, the states get plenty of leeway in where they place monitors, due to EPA’s desire to be flexible and mindful of the cost it imposes on localities. Recent research by Grainger, Schreiber, and Chang in 2018 shows that some monitors appear to be placed in areas of low pollution relative to elsewhere in the county, such as upwind of major point sources. Given the potentially high administrative cost to a local government of nonattainment status, and the high associated costs of coming into attainment for the region so classified, it would not be surprising to find that some local and state governments would be tempted to—or actually do—game the system, as to where they place monitors.
But statistical research cannot illuminate motive; research can only show that pollution hotspots are being missed, as Daniel and I show in a working paper, published in 2018.
Land-based monitors have another problem: they don’t all run all the time. Again, this problem is because of cost and, relatedly, old monitor technologies. Processing the data that monitors collect is expensive, and better technology comes with a high replacement cost. While new PM2.5 monitors tend to operate at least 300 days per year, we have found that 56 percent of PM2.5 monitors gathered data on fewer than 121 days in 2015, and 23 percent gathered data on fewer than 80 days. If these days were randomly distributed over the year, then setting the design value that characterizes air quality should not lead to bias. Unfortunately, the operating times of monitors are announced ahead of time. Because of the high cost to firms if their area is classified as nonattainment, and possible extra scrutiny from the local authorities if the firms are found to contribute to air pollution problems, firms have incentive to pollute more on days when the monitors are not operating. If air pollution “hangs around” for a few days, this strategy would not be particularly productive. But PM2.5 pollution can move quickly. And in fact, recent evidence from Zou in 2018 has shown that firms emit less on days when monitors measuring PM2.5 and PM10 (particles 10 microns or less in diameter) are in operation. These effects persist even after correcting for weekends and holidays.
Can We Fix These Problems?
Satellite data can help solve the problems that get in the way of accurately tracking air quality.
Satellites provide the spatial and temporal concentration detail that’s needed to reliably detect and monitor pollution on the ground. For example, sensors sent into orbit on satellites, such as NASA’s Moderate Resolution Imaging Spectroradiometer (MODIS), offer at least one concentration reading for a 1- to 3-km² grid over the entire country each day and at roughly the same time. And because those data are freely available (although processing the data has a high start-up cost), satellites eliminate the underlying cost and incentive issues that otherwise prevent a more comprehensive and appropriately placed land-based monitoring network from being developed and operated daily.
But are satellite data a panacea, and should they replace land-based monitoring in the designation of areas as attainment and nonattainment? The answer to both questions, in the short term, is no.
Let’s consider satellite data in a bit of detail. Satellites do not actually measure PM2.5 on the ground as such. They measure something called aerosol optical depth (AOD), which represents the density of aerosol particles. The measure is itself the difference between the solar radiation at the top of the atmosphere and the radiation that reaches the Earth’s surface. The more airborne particles there are, the less radiation is detected at the surface, and the larger is AOD. On cloudy days, no measurements are possible.
AOD must be converted to PM2.5. This is done using statistical methods combined with a global atmospheric chemistry transport model called GEOS-Chem (where “GEOS” stands for Goddard Earth Observing System). GEOS-Chem provides information about how pollutants are transported from one area to another by the wind, and how chemical compounds change as they travel. The resulting estimates are calibrated by lining up the estimated PM2.5 concentrations with the land-based readings. Thus, land-based monitors are critical inputs to the data conversion process.
In our study, Daniel Sullivan and I took PM2.5 estimates from satellite data for the grid cells surrounding land-based monitors and compared those estimates to PM2.5 readings from the monitors themselves. When we used PM2.5 concentrations calibrated for the entire globe, we observed serious errors and, more importantly, biases in the US satellite readings. Initially, we saw that PM2.5 concentrations from satellite data actually overestimated the readings from ground-based monitors when the latter registered high readings. However, using PM2.5 data calibrated only with North American monitors eliminated the bias, although the satellite readings became small underestimates of ground-based monitors with high concentration readings, and “small” errors around the true value remained.
Statistical research cannot illuminate motive; research can only show that pollution hotspots are being missed.
Thus, we recommend that satellite-based PM2.5 readings be used to supplement, but not replace, land-based monitors in the air quality designation process. Implementing a plan like this could also involve shifting the locations of land-based monitors to better measure pollution hotspots, installing new land-based monitors in areas that have none, and providing satellite readings of PM2.5 concentrations on days when the land-based system is not operating. For the last of these ideas, firms could be told that satellite data will be examined on such days; if hotspots flare up during the unmonitored periods, likely sources will be held to account. Of course, an easier (if costly) solution to the unmonitored-days problem is to replace the less frequently run monitors with devices that continuously operate. And cheaper still would be to keep secret the days during which the less frequently operating monitoring stations are scheduled to run.
While EPA has not yet embraced satellite monitoring data to supplement federally standardized monitoring, the agency has nonetheless embraced cheap sensor technology for the same purpose, as noted above. These devices are described in EPA guidance as useful for localities to “locate hotspots, identify pollution sources, and supplement monitoring data,” as well as provide more timely data. However, these supplemental monitoring devices generally perform very poorly, compared to data from monitors that operate by EPA’s federal reference methods. Satellite data could do better.
Setting Boundaries
We have left a fundamental and important issue for last: How should boundaries for nonattainment areas be determined, given that satellite data comes in at the relatively high resolution of 1- to 3-km²?
First, we need to understand how these boundaries are traditionally determined. Basically, states propose nonattainment area boundaries by combining contiguous areas that violate the standard, along with “nearby” areas that contain sources which might be leading to violations. States are required to use five types of information to propose these suggested boundaries: jurisdictional boundaries, air quality data, emissions data, geography and topography information, and weather data. States also use air quality modeling that shows where the pollution comes from and goes to. EPA is ultimately responsible for approving or setting boundaries of nonattainment, attainment, and unclassifiable areas.
Because pollution disperses, nonattainment boundaries work best when they err on the side of being more geographically expansive, rather than precisely drawn. High-resolution satellite data could provide for more tightly drawn boundary estimates, which may cut abatement costs in the long run, but erring on the side of public health with larger area boundaries seems wise, in general.
Still, EPA has ample opportunities to accept satellite data as a supplement to land-based monitor data in making decisions about attainment borders. Notably, adding satellite data would necessitate a change in EPA rules. Because of the high resolution of satellite data, their use would make clearer which jurisdictions should be included as violating. But these data—and indeed, any monitoring data—need to be supplemented with other types of data and air quality modeling to most effectively identify areas that contribute to air quality violations and which, therefore, are areas that should be included within nonattainment boundaries.
None of these methods is perfectly reliable, and particularly not on their own. But satellite data can provide an indispensable—and inexpensive—supplementary source of air quality data that can check against cheaters and weak analyses. By combining land-based data with satellite monitoring data, we can have much more confidence that our communities get an accurate gauge of their local air quality and are, therefore, properly classified.